Sasha (Alexander) Gusev is a statistical geneticist and an Associate Professor of Medicine at Harvard Medical School and the Dana-Farber Cancer Institute. His work involves the development of statistical methods for making sense of disease mechanisms and heritability from Genome-Wide Association Studies. He blogs about the genetics of complex traits at The Infinitesimal and maintains a primer on molecular heritability.
Awais Aftab is a psychiatrist in Cleveland, OH, and clinical assistant professor of psychiatry at Case Western Reserve University. He is interested in conceptual and philosophical issues in psychiatry and writes online at Psychiatry at the Margins.
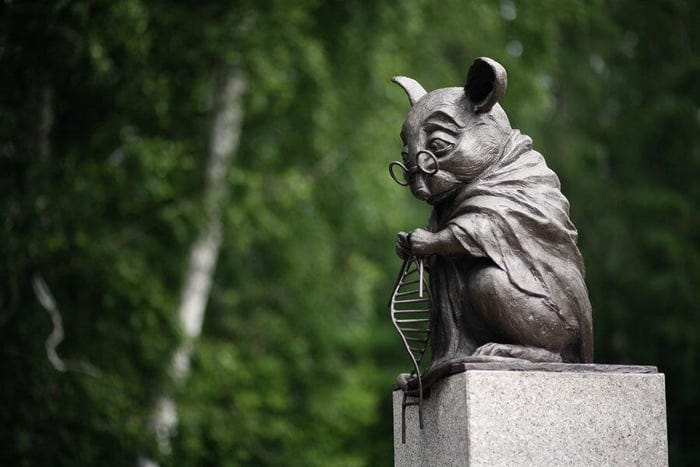
Aftab: Sasha, your blog, “The Infinitesimal,” is among my favorites on Substack and it’s a delight seeing you unpack complicated issues in genetics in your posts. I’m glad to have you for this Q&A to give readers a critical introduction to behavioral genetics (and I hope to follow this up with a Q&A with Eric Turkheimer, whose book you reviewed recently).
Gusev: Thank you for having me! I just want to start off by saying that I am a big fan of Psychiatry at the Margins, and it was one of the first blogs that got me thinking seriously about writing online. It is a fantastic example of how informal academic writing can enable one to think through complex and controversial concepts in a collaborative way. As I read more, I also realized that a lot of the specific issues related to nosology, diagnosis, and mechanism are extremely important outside of psychiatry, and, in many cases, we geneticists are only just coming to appreciate them.
Aftab: This means a lot, Sasha; I am grateful. Let’s start with the notion of heritability. Heritability refers to the degree of variation in a phenotypic trait in a population that is due to genetic variation between individuals in that population. This is not an intuitive concept for many people, and it’s common to see heritability being erroneously equated with “genetic causation.” If heritability doesn’t tell us about the degree of genetic causation, then what does it really tell us? If a condition has a high heritability, say 80%, what can we say about the role of genetics in this condition based on the heritability statistic alone?
Gusev: Heritability is a tricky parameter because it involves two unintuitive concepts: variance and ratios. A trait can be strongly driven by genetics but have zero heritability because there is no genetic variance in the population being studied. For instance, having two arms or two eyes is strongly determined by genetic mutations but these mutations are fixed in all humans, so there is no genetic variance to measure. Any phenotypic variance is driven by environmental factors like accidents (people losing an arm or an eye). So, even though these traits are genetic in origin, their heritability is effectively zero. Likewise, certain traits can have so little phenotypic variance (say, the roundness of your blood cells) that even with high heritability, the underlying genetic causation is actually very weak, only changing cell shape in minor ways. Moving away from these edge cases, there are a few ways to think about heritability more broadly.
First, heritability can define the expected change in the phenotype due to one generation of selection in a breeding experiment (typically quantified in The Breeder’s Equation). If you select tall animals for breeding, heritability will tell you how tall their offspring are expected to be in a fixed environment: the higher the heritability, the closer to the selected parental height. This is perhaps the most direct and practical definition of heritability, but it is also uninterpretable in humans because we do not satisfy the constraints of a breeding experiment (no matter what people may tell you): parents are not selected, mates are not paired up randomly, and the environment is constantly changing. In fact, while the Breeder’s Equation has been very effective in agriculture, in natural populations (animals experiencing natural selection in the wild), it often makes predictions that are inaccurate or even opposite to what is actually observed in the next generation.
Second, heritability can mean how much of a trait we can predict from genetic differences. This is the way the term has been used in the context of molecular genetics and Genome-Wide Association Studies (GWAS) up until 2018 or so (sometimes referred to as SNP-heritability or h2g). Given enough training data, 80% heritability means we can build a genetic score that predicts 80% of the trait variance (technically, produces a squared correlation of 0.8 with the trait). Because this is a predictive definition, it can include the contribution of non-causal genetic variables, for example, genetic variation that influenced traits in prior generations and was then passed down culturally through families (I’ll refer to this as “cultural transmission”). For some traits, the proportion of “heritability” that is actually operating through environment or cultural transmission rather than genes can be substantial, complicating the interpretation.
Third, heritability can define the expected change in the trait from hypothetically altering a person’s genes in their existing environment. Imagine flipping a specific genetic switch at conception and seeing how the person’s traits would differ: the higher the heritability, the larger the expected difference. This is sometimes referred to as “direct” heritability, and it is typically approximated in family-based analyses (e.g., “family GWAS”). This is probably the view of heritability that most people have in their minds, but it is also the most difficult to estimate in a truly unbiased manner. In fact, we currently have no way of reliably estimating direct heritability, though we are getting closer.
Even for traits with high direct heritability, the mechanism by which genes operate may not be biological in the way most people think, because genes are still mediated by the environment. In a society where opportunities, like education, depend on skin color, the heritability of education might seem high because skin color is highly heritable. But this is not genetic causation in a biological sense—it merely reflects the structure of society. In a different society without discrimination, the same exact genes would have no impact on education. Many human traits are an interplay between genes and environment, and heritability does not tell us the mechanisms through which a trait actually varies nor how it would vary in a new environment.
Aftab: Laypersons reading about heritability based on twin studies encounter a dizzying range of strong opinions. On one hand, some folks consider the twin heritability estimates to be some of the most robust and well-replicated body of literature in behavioral sciences, while others think that the methodology of many classic twin studies is so shoddy and problematic that nothing meaningful can be inferred at all. What would you say is the contemporary scientific assessment of the soundness and rigor of the classic twin-heritability literature?
Gusev: This has been a highly contested question and has become even more contested with the development of new methods for interrogating the role of genetics in humans in the past few decades. I should first say that we have little reason to doubt the raw numbers estimated by twin studies: the phenotypic correlations between monozygotic and dizygotic twins. Twin study research, like pretty much every other scientific field, went through a phase of data fraud and manipulation (for example, the Cyril Burt adopted twins scandal), but modern studies typically involve data from large-scale registries and rigorous analysis, and in that sense, they are well-replicated. The key question is how to interpret these correlations. I would also say that most geneticists now agree that nearly all measurable traits are directly influenced by genetic variation to some extent (i.e., have some non-zero direct heritability), which we can now observe at the level of individual genetic variants and in within-family GWAS that account for many biases. This point—just because parents with depression have kids with depression does not mean that the former entirely caused the latter—is an important caveat that twin studies and behavior geneticists have gotten right. What is contested is to what extent and for what traits are the twin estimates largely free of bias. On the one hand, classic behavior geneticists often conclude that the assumptions underlying twin studies are reasonable and that the resulting estimates can be treated as approximately correct. This position typically relies on the absence of evidence of bias, but cannot definitively rule bias out. On the other hand, there is growing evidence from molecular genetics and GWAS (i.e., actually measuring genetic variation and tracking how it correlates with phenotypes) that twin heritability estimates are inflated relative to what is observed with molecular data in unrelated individuals (the “missing heritability” problem I’ll talk about more below). However, these molecular studies often have their own assumptions or do not measure all available genetic variation or all traits, so they have not fully closed the case either.
Overall, I would say that there is broad consensus that all/most traits have some genetic component and that researchers should seek out genetically informed designs before concluding that something is caused by the environment. But it is also important to keep in mind that traits can be passed down culturally—you speak the same language as your parents because they taught it to you, not because you both share “same language” genes—and this cultural transmission can look a lot like heritability. There is also broad consensus that the numbers that come out of twin studies are subject to assumptions that are difficult to test and have, in some cases, been clearly proven wrong (for example, see Robinson et al. 2017 Nature Genetics, which includes a Who’s Who of geneticists in the author list and concludes that twin study estimates are inflated for BMI). In my personal opinion, the potential sources of bias in twin studies are so substantial and difficult to measure that twin estimates have very little practical value other than reiterating a basic fact we already know: most traits are under some genetic influence.
Gusev: There is broad consensus that the numbers that come out of twin studies are subject to assumptions that are difficult to test and have, in some cases, been clearly proven wrong.
Aftab: You’ve written that twin heritability models “can tell you whatever you want to hear” and that “The Classical Twin Design seems so simple—just compute two correlations and take the difference—but this merely is a consequence of assuming away all of the complexities.” Can you briefly explain what these complexities are?
Gusev: The classic twin study intends to measure the direct influence of genetics on a trait in a homogenous environment, but the real world is neither additive nor homogenous. When these deviations from the modeling assumptions occur, the twin model still has to assign the variability somewhere, which will induce bias. Specifically, if the influence of genes changes in the context of different family environments (a gene-by-shared-environment interaction), then the conventional twin model will simply assign all of these interactions into its heritability estimate. If the effect of genes changes in the context of other genetic variants (a gene-by-gene interaction), the twin model will likewise count these interactions as heritability. Such gene-gene interactions can arise in ways that are not very intuitive. For example, if you have multiple different heritable “pathways” by which a condition can develop, and it will develop if any of them are faulty (a kind of “weakest link” model), that will manifest as genetic interactions (see Zuk et al. 2012). Lastly, if spouses pair up and have offspring based on their shared phenotypes (assortative mating), for example, wealthy men marrying wealthy women, then the relatedness assumptions in the twin model may be incorrect, and this will also introduce a bias. The direction of this bias depends on the structure of the assortative mating, whether it is occurring on the trait itself or on background social factors (wealthy families marry wealthy families). There are even more complex gene/environment interactions (including interactions between interactions) that can bias the twin study estimates in either direction, but they follow similar principles.
Gusev: If the influence of genes changes in the context of different family environments (a gene-by-shared-environment interaction) or in the context of other genetic variants (a gene-by-gene interaction), then the conventional twin model will simply assign all of these interactions into its heritability estimate.
All of the above are real processes, and there may be ways of “correcting” the twin estimate if we come to understand them, but there is also a more basic methodological assumption: that monozygotic twins experience the same trait-influencing environment as dizygotic twins (the “equal environment assumption”). If the equal environment assumption is violated, for example if identical twins are treated more similarly by their parents or their community, then the estimate that comes out does not really represent anything interpretable anymore—the model is just broken. Guaranteeing the equal environment assumption is difficult because we typically know very little about which environments influence traits in general. In the post you linked, I discuss various studies demonstrating violations of nearly all of these assumptions for some traits. Which brings us back to the question of when twin models are wrong, whether we can correct their estimates, and what their wrongness tells us about the influence of various interactions.
Aftab: What’s your personal take on the problem of “missing heritability”? What’s the best way to conceptualize the problem, and what answers seem most likely?
Gusev: The missing heritability problem has historically referred to a few different discrepancies in molecular and twin/family models. Initially, GWAS of common traits were identifying very few associations, and people began to propose theories that maybe traits were not influenced by GWAS variants at all. These theories were incorrect. As GWAS sample sizes grew, it became clear that a substantial fraction of heritability was actually distributed across thousands of variants, each with very small effects. However, this soon revealed a second missing heritability problem: even if you add up the effect of every single variant in the GWAS (including those that are not individually statistically significant), they typically still do not come close to the heritability estimate from twin studies. Interestingly, the gap is often the largest for behavioral and psychiatric traits, precisely where one might expect environmental interactions. To give you an example, (Cheesman et al. 2017) estimated the twin heritability and GWAS heritability of a number of behavioral traits in the same individuals (twins that had also been genotyped), so cohort definition or measurement error were a non-issue. For teacher-reported ADHD, the twin heritability estimate was 69% while the GWAS-based heritability estimate was just 5%; with similar gaps for other behavioral traits. These are huge differences!
If we believe the twin study estimates, then this gap implies that there is a lot of causal genetic variation out there that GWAS/molecular data is not picking up. One way to think about this is that traits that are under stronger natural selection will have more of their genetic variants driven to low frequency, and thus less detectable by GWAS. So a big gap between GWAS and twins could imply that rare variants are very important due to strong selection. On the other hand, if we are skeptical of the twin study estimates, then this gap implies a substantial contribution from those environmental complexities I talked about previously. For a long time, the field of molecular genetics was operating under the assumption that the missing heritability was largely in the rare variants we had not yet measured. But a number of recent advances have started to tip the scales against that argument. First, some of the earlier molecular heritability estimates were found to be inflated by some mix of technical issues and cultural transmission, so the amount of missing heritability actually increased. Second, a new model was developed that could estimate total direct heritability using molecular data from mother-father-child trios, with very few model assumptions (the title literally states “… without environmental bias”; Young et al. 2018), and it too found estimates that were substantially lower than twins on average. Third, several studies have now actually measured the influence of rare variants in various forms, and they are so far not adding up to explain as much as we would expect from twin heritability estimates. Fourth, there is little evidence of the strong natural selection that would be needed to generate a massive trove of rare variants untagged by GWAS. I am a molecular geneticist, and this drumbeat of evidence from molecular data has convinced me that twin studies are either 2-3x inflated or estimate something fundamentally different from direct heritability.
What could explain the gap? All of the twin study biases I described above are likely at play to some extent, but my personal view is that interactions between genes and the shared/familial environment play a major role. I alluded earlier to a study of gene-environment interactions for BMI; there is also striking evidence for an interaction between educational attainment and socioeconomic status (SES), where the heritability drops substantially for individuals in high SES versus low SES environments (curiously, the opposite has been observed in twin studies). My guess is that hundreds or even thousands of interacting environments accumulate over a person’s lifetime, many of which are not even measurable. When we then study unrelated individuals in GWAS, the participants are experiencing all of these diverse environments, and so genetic variation has a weak influence on their outcomes. But when we look at twins, who share their rearing environment extremely closely (are literally born at the same time), all of these interactions get assigned to and inflate the genetics/heritability bucket.
Gusev: I am a molecular geneticist, and this drumbeat of evidence from molecular data has convinced me that twin studies are either 2-3x inflated or estimate something fundamentally different from direct heritability… my personal view is that interactions between genes and the shared/familial environment play a major role [when it comes to “missing heritability”].
The upshot of this whole debate is that much more rare variant data is now being collected, which will provide us with the ability to draw even stronger conclusions about the source of the missing heritability. I personally hope that the collection of diverse environmental measurements also keeps up so we can look for interactions too. The next few years will be an exciting time.
Aftab: As an outsider to the genetics field peeking in, it seems to me that we are uncovering more and more ways in which GWAS associations can arise—and be replicated—for environmental and cultural reasons rather than direct genetic ones. Is that so? And what does it mean for the future of GWAS?
Gusev: Absolutely, and it is good to keep in mind that just because your statistic replicates does not mean it is measuring what you think it is measuring. In GWAS this can happen either because genetic variants become confounded with cultural transmission: many generations ago a genetic variant had an influence on the trait, the effect of which was then passed down and amplified through generations of parenting and assortative mating to become correlated with the same trait in offspring today. Or because slight differences in variants between close populations (e.g., within countries) become correlated with trait influencing environments, and GWAS is sufficiently sensitive to pick these up as associations. What has been particularly surprising is that these sources of confounding can appear to replicate in studies that are completely independent or even conducted in different countries.
This means that for some traits we should be cautious that the individual effects being estimated are truly genetic in nature, even if they replicate, and especially cautious about polygenic scores that aggregate many such effects and can greatly amplify the confounding. That said, in cases where all we care about is predictive ability, the environmental confounding may be tolerable or even an advantage. If I want to identify individuals that are at high risk for schizophrenia for watchful waiting, it might be fine that my “genetic” score is capturing some predictive signal that is actually explained by the family environment these individuals were raised in. But we should be careful not to interpret these scores as coming strictly from genes.
The good news is that the field now has statistical tools to, at minimum, detect the presence of biases, typically by contrasting estimates from population-scale and within-family studies. We can quantify how much of the predictive heritability is driven by confounding factors, and if it is low, we can proceed with interpreting the GWAS results in the more conventional direct way. For many basic traits like height, this does indeed appear to be the case, and the standard assumptions of GWAS generally seem to hold. On the other hand, confounding is substantial for any trait having to do with education or socioeconomic status, for reasons that are not yet fully understood but that likely have to do with the complex environmental interactions, assortative mating, and stratification those traits experience. It is unknown to what extent this is the case for rarer psychiatric conditions for which we need to collect more data, but that data is being collected. Even for traits that are confounded, we will also be able to estimate something approaching the direct genetic estimates as the family GWAS studies get larger. So, at the moment, there are ways to detect bias in GWAS, and in the future we may even be able to correct for it.
Aftab: If the heritability of a trait behaves differently within versus between families or across environments, what does that tell us about the trait?
Gusev: I find this to be one of the most fascinating observations from recent genetic studies. I mentioned previously that genetic influences can become confounded through cultural transmission over generations. In family GWAS, we can estimate both the “direct” effects acting in you, as well as the “indirect” effects acting through cultural transmission or confounding. For some traits, such as educational attainment, it turns out that the direct (within-family) effects are much weaker than the total (between-family) effects. The typical genetic scores and estimates between families are thus capturing a substantial amount of external environmental influence in some form. For such traits, we may be motivated to look closer at the influence of parental/nurturing or family environments, or fine-scale geographic clustering/confounding.
Gusev: For some traits, such as educational attainment, it turns out that the direct (within-family) effects are much weaker than the total (between-family) effects. The typical genetic scores and estimates between families are thus capturing a substantial amount of external environmental influence in some form.
Even more mysterious is the observation from several recent studies that indirect effects (ostensibly from parents) actually appear to be negatively correlated with the direct effects. This is very surprising. The plain interpretation is, for instance, that the genes that increase depression in you are also acting in your parents to decrease your depression through nurturing (and by the way, that is a real finding from Cheesman et al. 2020). This could be a real biological effect, which would imply that the mechanisms of cultural/nurturing influences can be very different from those of direct biological influences. To speculate a little, perhaps factors that increase depression and anxiety in you also cause you to identify and mitigate it in your children. It would also mean that we need to consider the influence of genetics on a given individual within their specific nurturing context. Alternatively, this negative relationship may be explained by statistical biases having to do with the way studies are ascertained; meaning that the participants in these genetic analyses are fundamentally unusual relative to the population. Whatever the cause, it tells us that the trait has a complex relationship with its environmental and societal context.
Aftab: There is a great deal of pessimism about genetics in psychiatry and clinical psychology. Many practitioners see the findings of GWAS to be highly underwhelming, and they can’t imagine how devoting further resources to studying genetics at the expense of other scientific programs can deliver meaningful results when the conditions are highly polygenic, the individual genetic associations are nonspecific in nature (and perhaps confounded by gene-environment interactions in complex ways), and psychiatric polygenic risk scores—with perhaps the exception of schizophrenia—explain such small proportions of liability that any practical application seems unlikely. Is such pessimism well placed? What room for optimism is there?
Gusev: It is worth acknowledging that certain traits show substantially lower predictive accuracy than we had originally expected from family/twin studies. For schizophrenia, which was thought to be largely genetic, the most we can expect from a common variant polygenic score is an accuracy (R-squared) of ~0.24, with the current score reaching about a third of that (Trubetskoy et al. 2022); for major depression disorder, the most we can expect is an accuracy of 0.087 (Wray et al. 2018). Even for these estimates, we do not yet know to what extent they may be inflated by the kind of stratification and confounding I’ve mentioned. At this level of predictive accuracy, the genetic predictors are probably not game-changing, but I believe they can still play a significant role in a broader integrative predictive model (similar to the way that polygenic scores for cancer risk are now becoming a component in models that incorporate other lifestyle and demographic factors). Since GWAS is relatively cheap and reliably produces results, I expect that the sample sizes will keep going up until these accuracies hit their max. But if we look beyond prediction, there are reasons for genuine optimism.
Most importantly, heritability does not tell us how modifiable a given trait is, and this goes both ways: just as high heritability does not mean we are all forced to follow a path defined by our genetics, low heritability does not mean that we cannot identify biologically meaningful mechanisms that could lead to important interventions. GWAS can point us to genetic variants that have small effects in the general population but could have large effects when targeted and amplified through treatment. One example is the recent discovery that rare genetic variants in the CHRNB2 gene are associated with a whopping 35% lower odds of smoking (Rajagopal et al. 2023). In mice (with all of the caveats that implies), knocking out this gene essentially seems to make smoking feel less pleasurable and addicting. Although very few people carry these variants, such that the predictive effect is negligible in the population, inducing this effect in all smokers with a therapeutic would have a massive reduction effect in the population. Identifying just one such drug target for a common psychiatric disorder would justify all of the psychiatric GWAS investments, in my opinion.
Gusev: Low heritability does not mean that we cannot identify biologically meaningful mechanisms that could lead to important interventions. GWAS can point us to genetic variants that have small effects in the general population but could have large effects when targeted and amplified through treatment.
There is also still much to be learned about basic trait biology. We can find traits that share genetic mechanisms with psychiatric disorders even if they are not measured in the same individuals to get a better understanding of shared biological pathways (genetic correlation). We can use genetics to test whether a given exposure may causally increase or reduce the risk of a psychiatric trait, by using genetic influences on the exposure as a kind of randomization (Mendelian randomization). We can identify individuals that are at an extreme genetic score value for a condition but did not develop is, and investigate whether any environmental exposures may have compensated for their risk (resilience). And, of course, there is the big question that much of human genetics is trying to understand: whether the thousands of polygenic effects converge on a smaller set of biological “pathways” or “programs” that are more directly interpretable.
Aftab: How has your thinking about behavioral genetics changed over the course of your academic career?
Gusev: For much of my career, I thought that the problems of confounding in GWAS analyses were largely solved by standard methods. Now that we have had several well-powered within-family studies, I have been genuinely surprised by how much confounding is not, in fact, being controlled, particularly for behavioral phenotypes. I think these issues are very important for geneticists to grapple with as studies are getting larger, more heterogenous, more complicated, and their results are being more aggressively interpreted (or misinterpreted) by the public. On a positive note, the fact that we can’t seem to get rid of the environment from our analyses also means there may be more opportunities for environmental interventions and risk factors than we had initially thought.
Beyond GWAS, I have become much more sensitive to the way statistical simplifications that are intended to make modeling the world easier can go on to dominate how we think the world actually functions. Heritability is one such concept, which hides a lot of the underlying complexities in a single number, and that number often gets misinterpreted further as an estimate of genetic causation. Factor analysis and clustering are other concepts/tools that were intended to represent data in compact ways but gradually become interpreted as biologically real. The ongoing debate over the validity of the “p-factor” (or general psychopathology factor) is one example, as are similar debates over the general factor of IQ tests. The interpretation of genetic ancestry components as evidence for “biological races” is another example. Even terms like “pathways” and “programs” that I’ve used above now raise some trepidation in me: do we really know what a biological pathway is or are we just relying on the abstractions that are currently available to us? Don’t get me wrong, I am still a strong proponent of statistical modeling, but I’ve grown much more aware of how models and parameters can create their own reality.
Aftab: Thank you!
This post is part of a series featuring interviews and discussions intended to foster a re-examination of philosophical and scientific debates in the psy-sciences. See prior discussions here.
See also:
Thank you for another educational and thought-provoking article. Dr. Gusev notes, “for schizophrenia, which was thought to be largely genetic, the most we can expect from a common variant polygenic score is an accuracy (R-squared) of ~0.24, with the current score reaching about a third of that (Trubetskoy et al. 2022).” But if the phenotype we call schizophrenia is really a wide spectrum of disorders with similar symptoms, might the R-squared be higher if we identify the different conditions that we today broadly call schizophrenia and look at them separately?
Because this is a predictive definition, it can include the contribution of non-causal genetic variables, for example, genetic variation that influenced traits in prior generations and was then passed down culturally through families (I’ll refer to this as “cultural transmission”). For some traits, the proportion of “heritability” that is actually operating through environment or cultural transmission rather than genes can be substantial, complicating the interpretation.
Does this imply a biological/genetic "start" that over time, transforms into a non material or less biological cause for a particular behavioral trait of interest? Hopefully that question makes sense. What would be an example of this?